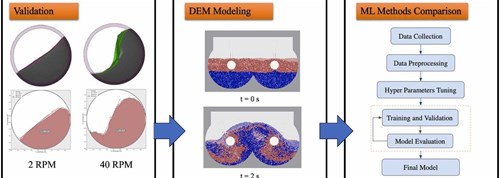
Papers
Analysis of cohesive mannitol particle mixing: A comparative study of machine learning methods
Behrooz Jadidi, Mohammadreza Ebrahimi, Farhad Ein-Mozaffari, Ali Lohi, Aurélien Neveu, Filip Francqui, Analysis of cohesive mannitol particle mixing: A comparative study of machine learning methods, Chemical Engineering Research and Design, Volume 214, 2025, Pages 188-201
Abstract
An in-depth analysis of the cohesive powder blending of Mannitol with different sizes within a double-paddle mixer was conducted, emphasizing the development of a robust quantitative simulation model via the Discrete Element Method (DEM). Leveraging datasets from DEM, the Random Forest (RF), Artificial Neural Network (ANN), and Multivariate Polynomial Regression (MPR) algorithms were employed to construct predictive models for the system's mixing index. Our key findings indicate that impeller speed and mixing time are the most critical factors significantly influencing mixing performance. Through a comprehensive comparison, RF demonstrated the highest accuracy in predicting the mixing index among the ML techniques tested. The study examined essential operational parameters—such as fill level, impeller speed, and particle characteristics—to assess their significant impacts on blending efficiency and product quality. This study contributes to the field by providing a calibrated DEM model and showcasing the effective integration of DEM with machine learning to predict and optimize mixing performance, thereby reducing computational efforts and enhancing industrial mixing processes for cohesive particulate systems.